Multi-Objective Optimization of MEMS Sensor Manufacturing Process with Multi-Sigma®
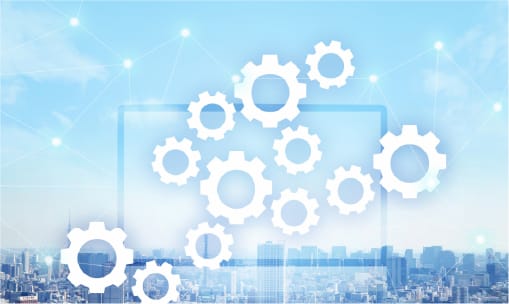
We used the AI analysis platform, Multi-Sigma®,
to predict and analyze factors and optimize the manufacturing of high-performance MEMS sensors
1. Prediction of performance indicators
(sensitivity, linearity, signal-to-noise ratio)
Multi-Sigma’s AI prediction function enables the training of an AI model using input data (explanatory variables) and output data (objective variables) to establish the relationship between them. This AI model can predict three performance indicators—sensitivity, linearity, and signal-to-noise (S/N) ratio—based on seven manufacturing parameters: etching time, etching temperature, deposition pressure, deposition temperature, deposition time, exposure amount, and development time.
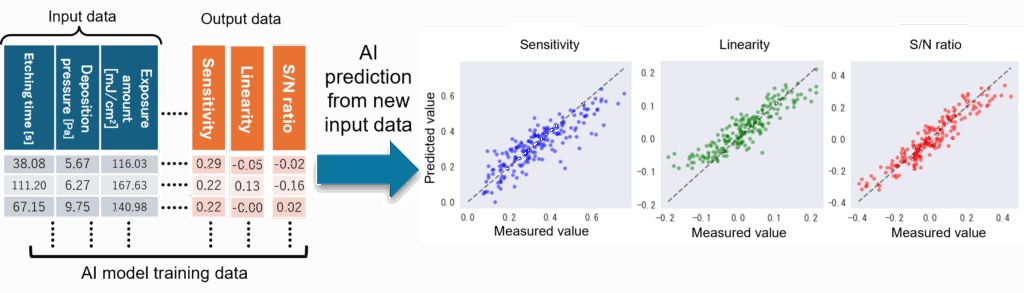
2. Factor Analysis of the Manufacturing Process
Multi-Sigma’s factor analysis allows you to identify manufacturing process conditions that positively or negatively impact the performance indicator.
Sensitivity: Deposition time (23.7%), Deposition temperature (18.0%), Etching time (16.5%)
Linearity: Etching Temperature (29.8%), Etching Time (29.1%), Exposure amount (12.9%)
S/N ratio: Development time (39.3%), Exposure amount (37.4%)
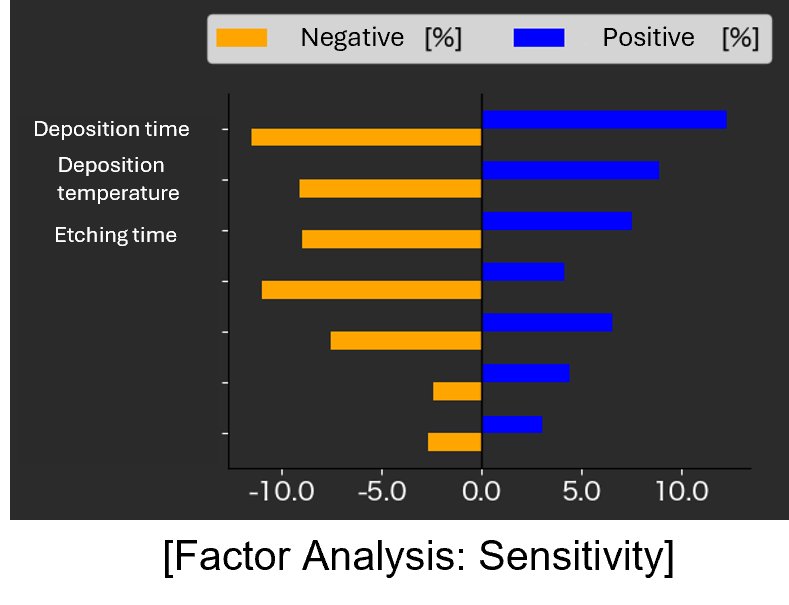
3. Optimization to maximize sensor performance
Multi-Sigma’s optimization suggests the optimal combination of manufacturing parameters to achieve the desired performance indicators.
Expected outcomes:
1. Improved efficiency of the manufacturing process
2. Reduced experimental cost
3. Shortened product development time
Advantages of Optimization with Multi-Sigma® :
Multi-objective optimization is achievable. By considering the interactions between objective variables, optimal manufacturing process conditions can be determined, even when conflicting effects exist. Additionally, the optimization can be customized to the defined scope of the manufacturing process.
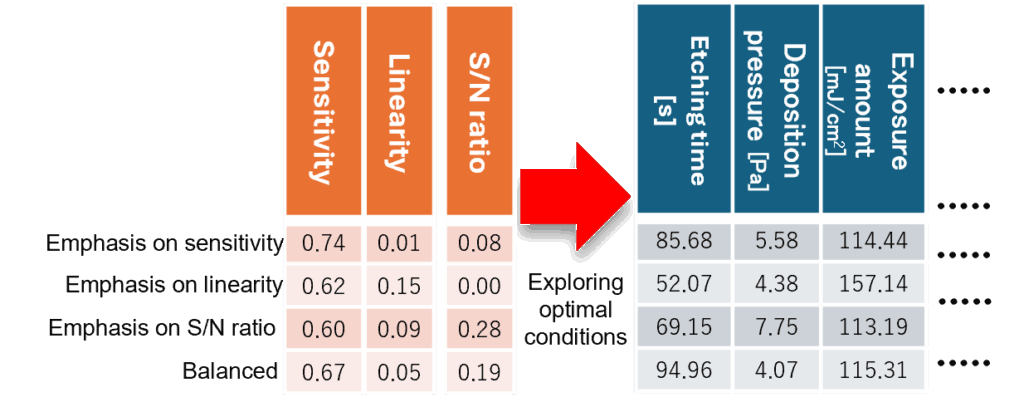
Note: The data used in this analysis is an artificial dataset that mimics actual data.