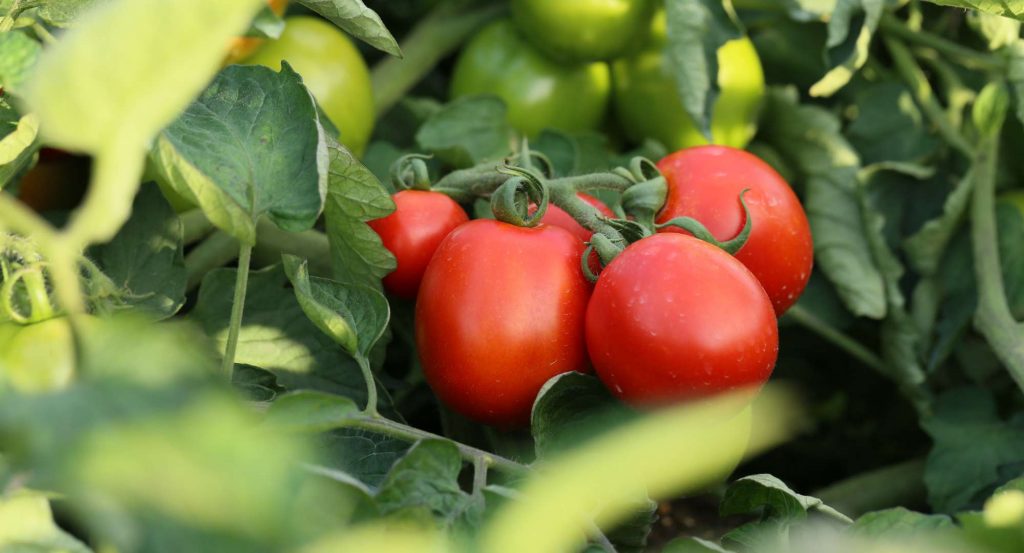
Utilizing AI In Smart Agriculture, Kagome Has Employed Multi-Sigma By Aizoth To Predict The Yield Of Fresh Tomatoes.
Kagome has aimed to enhance the accuracy of yield prediction, a vital factor in fresh tomato supply-demand adjustments, by developing a fresh tomato yield prediction system utilizing Multi-Sigma. In this conversation, we have the leader, Mr. Imamura, and Mr. Kawajiri, the developer from Aizoth Inc., who created Multi-Sigma, to talk more about this incredible development.
Company Name: Kagome Co., Ltd.
Business Activities: Manufacturing and selling of seasoning, preserved foods, beverages, and other food products; procurement, production, and sales of seeds and vegetables.
Revenue: ¥2,247 billion
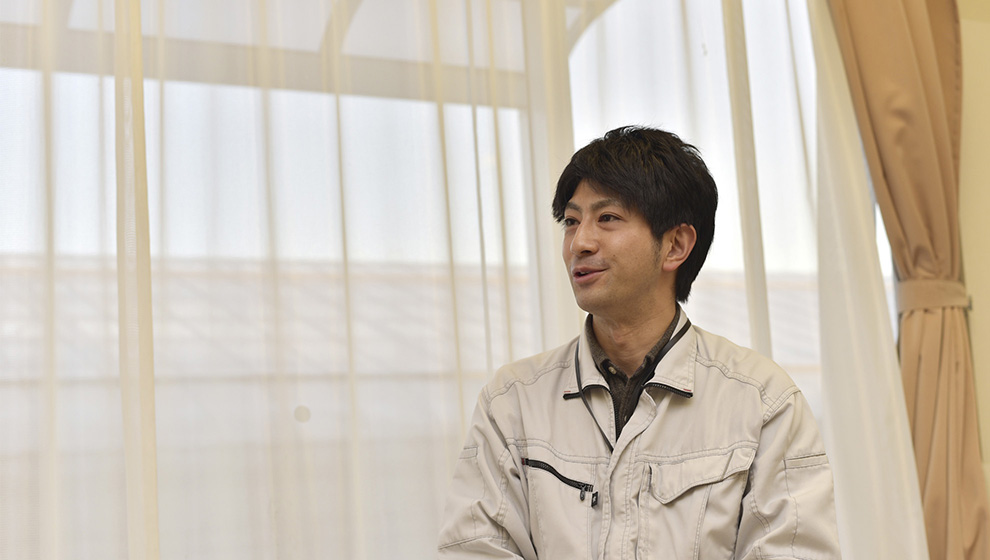
Challenges Before Implementing AI For Yield Prediction And Impact On The Field After Implementation
Kawajiri: “Thank you for joining us today. Could you please tell us about the challenges you faced before implementing the fresh tomato yield prediction?”
Imamori: “Certainly. In our fresh tomato business, where we handle everything from production to distribution and sales, having accurate information about the tomato yield, such as how much and when tomatoes can be harvested on the farm, becomes incredibly important. Previously, this yield prediction relied on the intuition and experience of the farmers. However, improving the accuracy of these predictions posed a significant challenge.”
Kawajiri: “I see. Could you share some of the trial and error involved in developing and implementing the prediction system?”
Imamori: “Our initial approach focused on using weather forecasts to enhance prediction accuracy, but long-term weather forecasting proved challenging, leading to a roadblock. We then shifted our focus to utilizing our vast data resources. Subsequently, determining how to process this data became crucial. We incorporated various statistical analysis and AI techniques, eventually concluding that Azoth’s’ AI was the way forward.”
Kawajiri: “While this prediction system seems to have been highly successful, what strengths of your company do you believe were leveraged?”
Imamori: “Given our involvement in the entire fresh tomato value chain, having timely information about tomato yield enabled our sales team to respond flexibly and take necessary actions, which I consider a significant advantage.”
Kawajiri: “What impact and effects do you think this system has had on your business?”
Imamori: “We believe it has had an impact across the entire value chain. For production, farm workers can now predict when and how much tomatoes can be harvested, leading to better adjustments in workload and staffing. Moreover, the more accurate yield predictions for distribution and sales enable strategic sales activities.”
Kawajiri: “What is the sentiment around the current prediction system?”
Imamori: “It’s progressing very smoothly. Recently, I spoke with someone at a farm who mentioned that “the AI predictions are more accurate than the traditional ones,” which further instills trust among field personnel.”
Kawajiri: “From the perspective of the farmers, introducing AI into long-standing human-based methods might have caused some apprehension. Do you think there is an aspect of AI being trusted only after using it?”
Imamori: “I believe so. It’s somewhat surprising to see how well this tool is performing. Without experiencing it firsthand, it’s hard for them to believe. The trust from the farms, which have been using it for a long time, is quite strong. The most challenging part is implementing it practically. We were fortunate to closely involve the users from the development stage. Every time we visited the farms, discussing how they could use it effectively, it became apparent that what we delivered was very user-friendly for them. While not all technologies yield such positive outcomes, this has been a very successful project for us.”
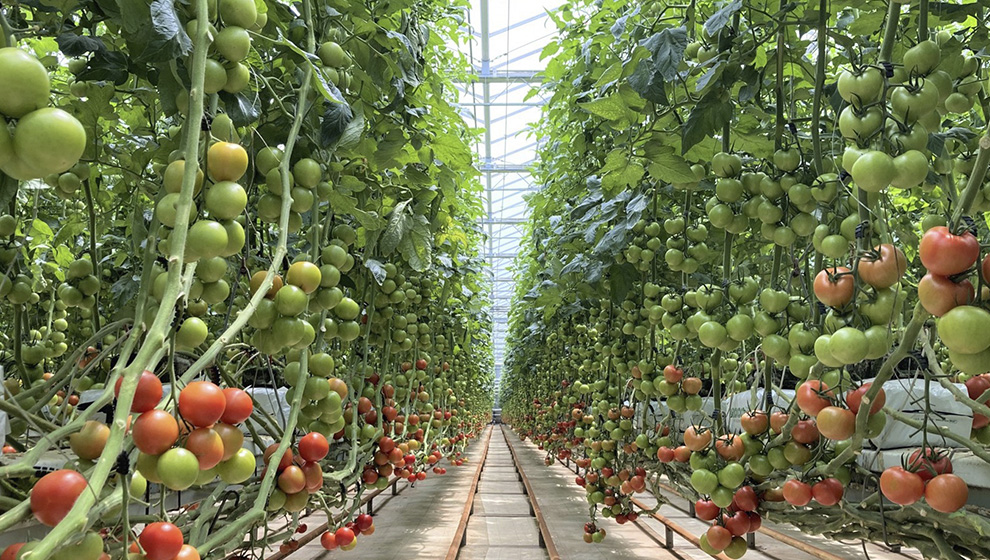
From A Proprietary Yield Forecasting System To The Utilization Of Multi-Sigma
Kawajiri: “How did our Multi-Sigma contribute to the yield forecasting system?”
Imamori: “For this yield forecasting, we utilized big data from past cultivation records. With the increasing climate variability, we realized the importance of incorporating not only historical data but also recent data to continuously update our prediction models. Being able to quickly update models and increase training data when data accumulates is a significant advantage of using Multi-Sigma. Furthermore, analyzing the factors contributing to the yield from the resulting model is expected to contribute to the future development of our cultivation techniques.”
Kawajiri: “Were there any distinctive aspects in how the data was collected?”
Imamori: “One thing I noticed from this experience was that the data collected at each farm was meticulous, and the data format was excellent. Although the data was originally collected for our cultivation guidance, the fact that such accurate prediction models could be developed from this data emphasizes the value of data. We’ve become more conscious of improving data accuracy by focusing on how data is collected. Ultimately, we’ve realized that data quality directly impacts our operations.”
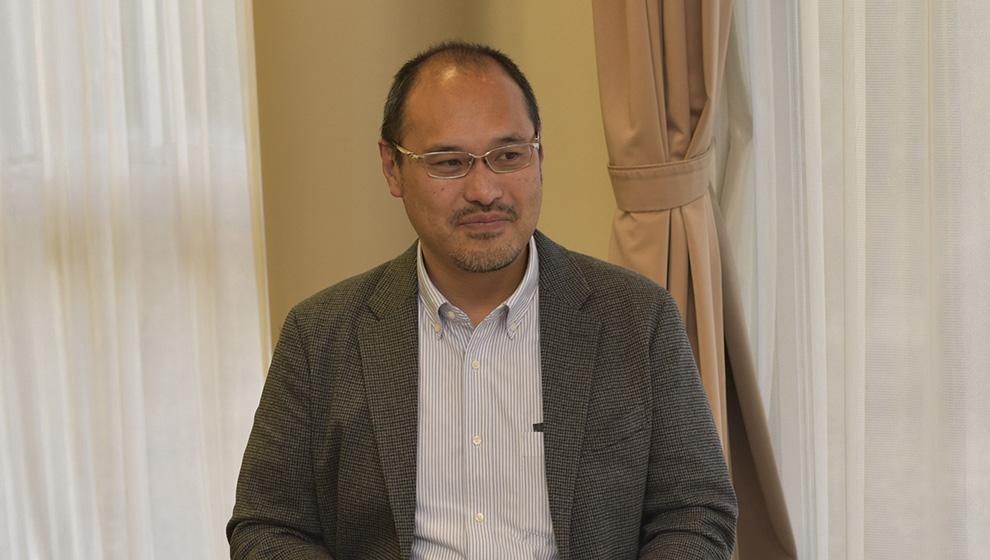
The Compatibility Between AI And Agriculture
Kawajiri: “In the process of data analysis using AI, have there been any changes or realizations for you, Mr. Imamori?”
Imamori: “I’ve been researching agriculture for a long time, and I’ve always felt that agriculture involves many complex factors. Even looking at just one output, it’s often difficult to understand or explain what factors are involved. However, by creating the yield prediction model and analyzing various data, I feel like I’ve been able to understand agriculture a little bit more. Conversely, using AI, I’ve come to realize that agricultural data is very suitable for AI.”
Kawajiri: “On the other hand, were there any points where you felt perplexed by the approach used in AI, as it’s not the conventional method for statistical analysis?”
Imamori: “When doing statistical analysis, I think there’s a need to be mindful of the correlation between parameters. However, with AI, I think it was beneficial that we didn’t have to worry too much about that. Also, actually using AI, I didn’t expect the accuracy to be as high as it was, and as we increased the training data, the accuracy visibly improved. So, I feel a great sense of scalability and potential, and I expect that we will also be able to use it in other ways in the future.”
Kawajiri: “What I’ve been feeling in recent years is that while AI tools are advancing significantly, there’s a part of the human side of mastering AI that can’t be taught like a textbook, and I personally think that might be a future challenge. What are your thoughts?”
Imamori: “Yes, indeed. Through our various discussions, Mr. Kawajiri, I’ve come to recognize the significant importance of this aspect of expertise. Having the tool is one thing, but together with that, how to make the data, how to handle calculations, and so on, it would be nice if there were a way to learn those together as a set.”
Kawajiri: “In the future, if there’s anything your company expects from us or from Multi-Sigma, could you please let us know?”
Imamori: “Certainly, I think Multi-Sigma is a very user-friendly analysis tool that’s easy to use without coding, so I’d like to continue using it more and more. The momentum to use it in our company is increasing. It’s all about ease of use. When people hear about AI, some may think, “It might be difficult to use,” so I think if it could be a tool that anyone can easily use, more and more users will come.”
Kawajiri: “Thank you very much. We’ll continue to strive to make the tool more user-friendly, including the UI, and easier to analyze. Thank you very much for the nice conversation.”