Virtual Experiments Using AI Chain Analysis to Support the Modularization and Systematization of Production Processes
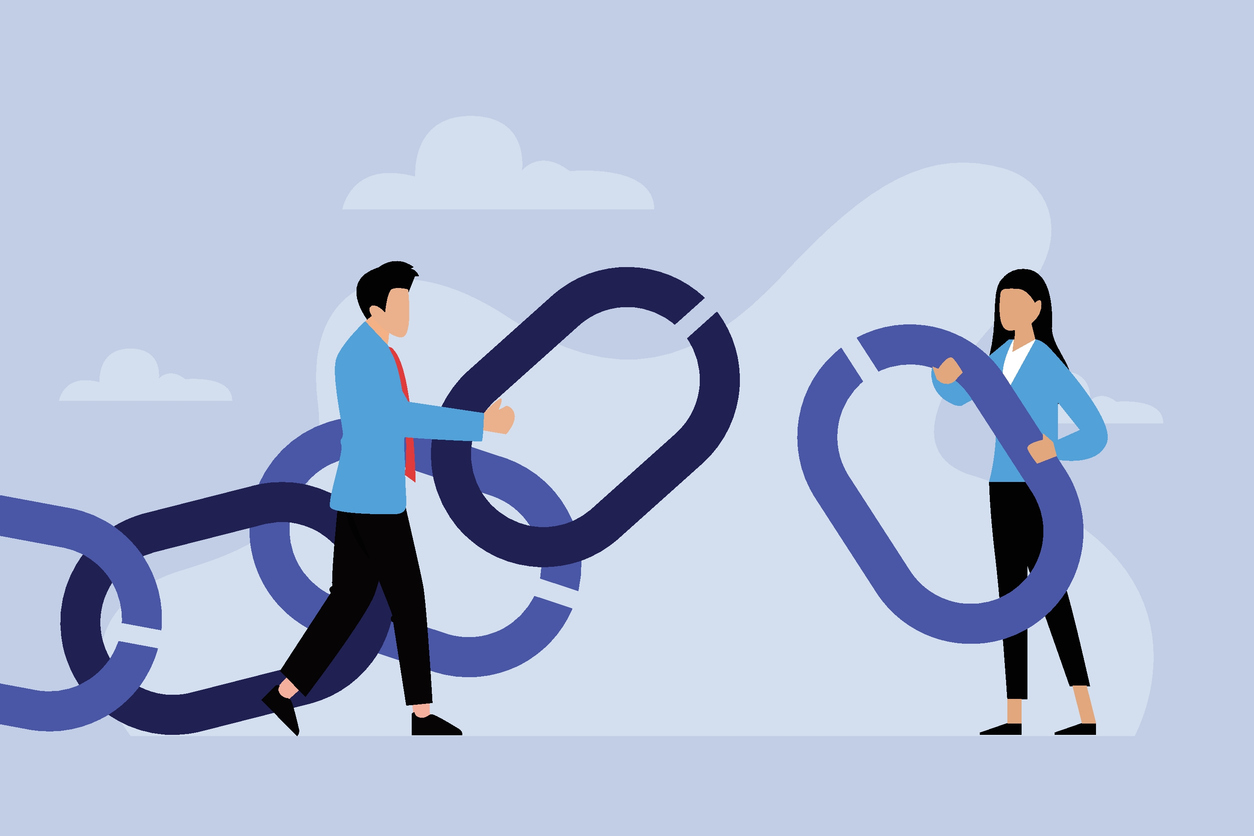
AIZOTH Inc. has developed an innovative AI chain analysis system, the first of its kind in the world. This internationally patented analysis system will be available on the no-code AI analysis platform, Multi-Sigma, starting July 31, 2024. In this article, we would like to introduce why AI chain analysis is necessary and how it can be used in the real world of R&D.
AI Analysis for Multi-step Production Processes
In the field of manufacturing, it is rare for a final product to be created through a single step process. Generally, a final product is completed through multi-step processes. For modelling multi-step production systems, the traditional approach has been to treat all the processes as a single ‘black box.’ The entire system’s inputs and outputs were combined into one experimental data set and used to train a single machine learning model (see Figure 1).
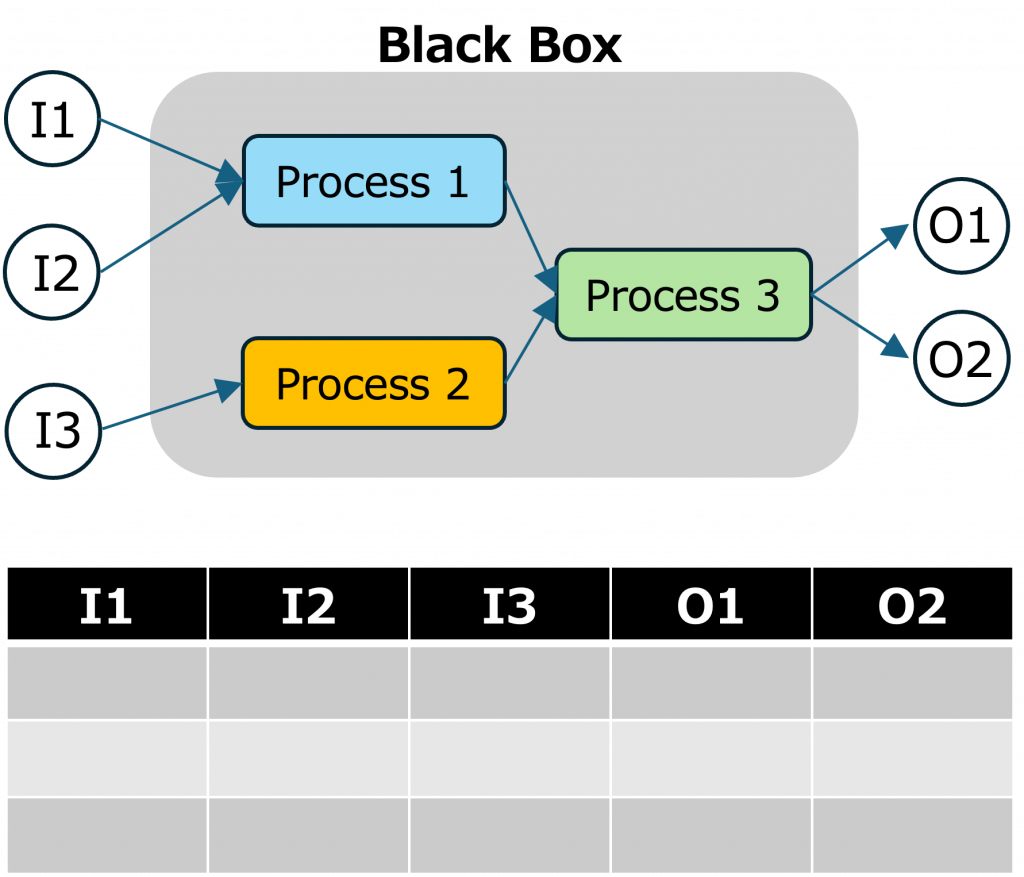
Figure 1: Black Boxing of Multiple Processes
Challenges with Existing Methods
While this black-boxing method has a simplified model and requires less time for analysis, it has several challenges. First, if even one step in the multi-step process undergoes changes in experimental conditions or procedures, the entire system’s experimental data must be recollected. As the system becomes more complex and larger in scale, the risk of having to redo experimental data collection due to process modifications increases significantly. Second, by treating multiple processes as a single black box, it becomes difficult to understand the internal workings of each process. If a defect appears in the final product, identifying which step caused the issue becomes impossible, making it difficult to implement improvement measures. Finally, if all processes are combined into a single experimental data set, experimental data cannot be consolidated until all systems are in operation, which makes it difficult to conduct experiments when the system is large.
Partitioning and Combining AI models
To solve the aforementioned problems, we first divide the multi-step process into individual steps, data collection at each step, create an AI model, and then built an AI chain analysis system that can analyze them as a single system by connecting them together (Figure 2). This chained system can be used to perform forecasting, factor analysis, and multi-objective optimization.
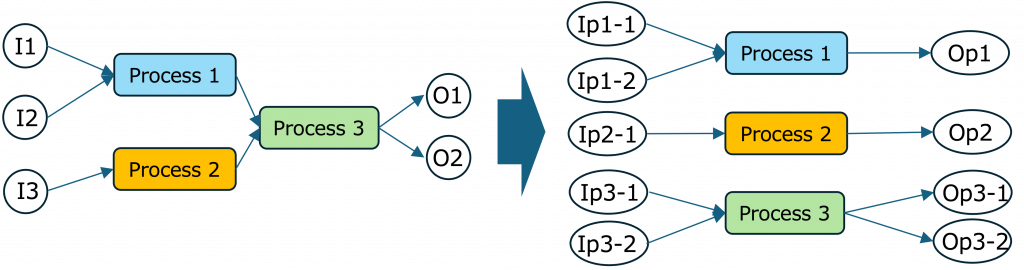
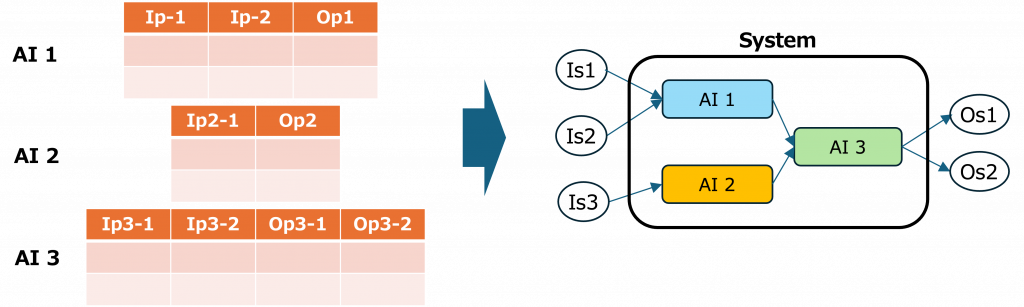
Figure 2: Chain analysis of multiple processes
Advantages of Chain Analysis
By creating separate models for each process, if ‘Process 1’ is updated and its output parameters are consistent with previous data, only the model for ‘Process 1’ needs to be adjusted. It can then seamlessly connect with the existing models for ‘Process 2’ and ‘Process 3’, removing the need to conduct experiments across the entire system again. In addition, since experiments can be performed for each process, there is no need to run the entire system to acquire experimental data (of course, it is also possible to run the entire system to acquire data for all processes at once). Furthermore, since factor analysis can be performed for each process, it is easier to understand the internal mechanisms of the system. Finally, and most importantly, by dividing the AI models based on each process, individual AI models can be easily adapted for use in other applications.
Utilize past AI models to represent complex processes
By reducing the size and simplifying the processes represented by each AI model, it becomes possible to share the same AI, which can then be combined to represent more complex systems. On the other hand, if complex processes are combined into a single model, it becomes difficult to standardize the AI and combine it with other processes. Like Lego blocks, by making the individual blocks simple and small, they can be combined to represent various forms.
Chain analysis will be needed in all fields in the future
This discussion of subdividing processes and then combining them into a system is now advancing in all fields in the form of modularization and systems for production processes. Modularization is a familiar term for mechanical systems such as automobiles, and in the semiconductor field, the terms “unit process” and “process integration” are used. In the field of chemistry, it has been systematized as a single area called process systems engineering.
To respond to these trends, AI chain analysis is an indispensable tool, and Multi-Sigma is the only tool in the world that can link AI models to perform prediction, factor analysis, and multi-objective optimization. We hope you will give Multi-Sigma a try and experience its benefits firsthand.