Smart Agriculture with AI at Kagome: Using AIZOTH’s Multi-Sigma to Predict Fresh Tomato Yield
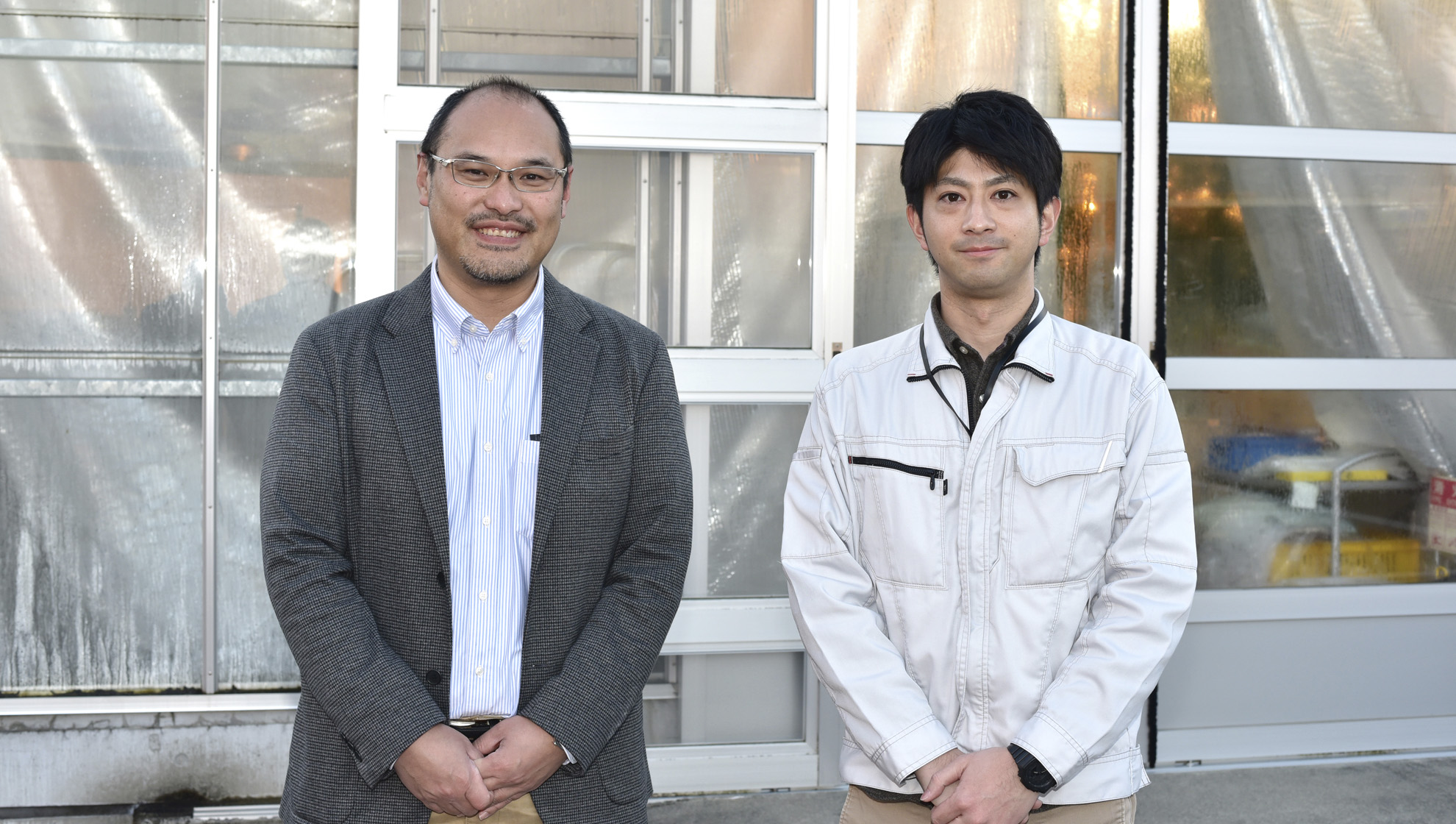
Kagome has developed a fresh tomato yield forecasting system using Multi-Sigma with the aim of improving the accuracy of yield forecasting, which is an important piece of information when adjusting the supply and demand of fresh tomatoes. We had a talk with Mr. Imamori, the leader of the system, and Kawajiri, the developer of Multi-Sigma, from AIZOTH Inc.
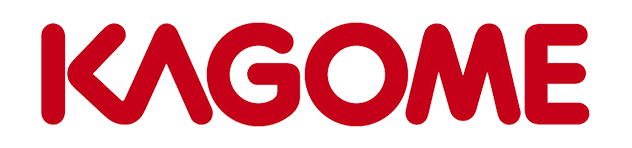
Company Name:
Kagome Co.
Business Lines:
Production and sales of seasonings, preserved foods, beverages, and other food products Purchasing, production and sales of seeds and seedlings, fruits and vegetables
Net Sales:
205.6 billion yen (fiscal year ending December 31, 2022)
Challenges before introducing AI for yield forecasting and its impact on the field
Thank you very much for joining us today. First of all, please tell us about the challenges you faced before implementing fresh tomato yield forecasting.
Yes, in our fresh tomato business, we handle everything from tomato production to distribution and sales, so it is very important to have yield forecast information on how much tomatoes can be harvested on the farm and when. Until now, yield forecasts were made by farm staff based on their intuition and experience, but the forecasts were not always accurate, and the issue was how to improve them.
I see. Could you tell us about the trial-and-error process you went through in developing and introducing the forecasting system?
Our first approach was to use weather forecasts to make yield predictions with high accuracy, but it was difficult to make long-term weather predictions, so we ran into some obstacles. Then we switched to an approach of utilizing the large amount of data we had on hand. After that, we asked ourselves how we could process the data, and as a result of incorporating various methods of statistical analysis and AI, we finally came to the conclusion that we would use AZOTH Inc. AI.
I think it can be said that this forecasting system was a great success.
Our company is involved in the production, distribution, and sales of fresh tomatoes, so I think the fact that our sales staff responds flexibly and takes action based on information on how much tomatoes were harvested was a very important point.
How has the newly developed system affected your business?
I believe it has had an effect on all value chains. First, on the production side, farmers can now predict when and how much tomatoes will be harvested. We expect that this will allow them to better adjust their workloads and staffing. In addition, we have been able to achieve more accurate yield forecasts for subsequent distribution and sales activities, and I think this will have a very significant effect on our strategic sales activities.
How are things going with the forecasting system these days?
Yes, it is going very well. I had a conversation with a vegetable gardener the other day, and he said, “There are many situations where the AI is more accurate than conventional forecasting. It seems that this has made the people in the field trust us even more.
From the viewpoint of people in the field, there was probably some resistance to the emergence of AI after many years of human-based work. Do you think that people trust AI only after they have actually used it?
I think so. I was surprised to see how well this tool works. I think it is difficult to get people to believe in a tool unless they actually feel it, and the vegetable gardeners who have been using it for a long time have a lot of trust in it.<br>There is a big hurdle in first creating the technology and then getting people to actually use it. It is important to be able to say that something like this has been developed, but the hardest part is actually implementing it. What was good about this project was that we were very close to the users from the development stage. We visited the vegetable garden each time and talked with Mr. Kawajiri about what kind of products would be useful, while developing the product. It is not often that all the technology we have developed comes to fruition, but this time we were able to do a very good job.

From a proprietary yield prediction system to using Multi-Sigma
How did Multi-Sigma help you in your yield prediction system?
This time, yield prediction is based on big data of past cultivation records. Recently, we have been facing a lot of climate change, so it is very important to update the forecasting model by adding not only old data but also recent data as needed. The big advantage of Multi-Sigma is that we can create models more easily to take actions such as updating the models and increasing the training data as soon as data is accumulated. I also believe that the analysis of factors that contribute to the yield from the obtained model will lead to the development of our cultivation technology in the future.
Was there a particular way of taking data?
One of the things that I thought was good about this project is that the data was collected in each garden and the format of the data was good. The data was collected for our cultivation guidance, and the fact that we were able to create such a highly accurate forecasting model from that data makes me feel that the data is a treasure trove. Now, in order to improve the accuracy of data, we are also becoming more conscious of the way we collect data. I feel that we have become more aware of the fact that the data will eventually come back to us.

Affinity between AI and agriculture
As you analyze data with AI, have you noticed any changes in your own sense?
I have been conducting research on agriculture for a long time, and I have always felt that there are many complicated factors in agriculture, and it is very difficult to understand or explain what is involved in even a single output. However, by creating this yield forecasting model, we were able to obtain a variety of data and analyze them, and I feel as if I have been able to decipher something about agriculture. Conversely, by using AI, I have realized that agricultural data is very suitable for AI.
On the other hand, did you feel puzzled by the fact that AI is a non-traditional approach to statistical analysis?
I think it was good that we could use AI without worrying too much about the correlation between parameters, whereas we used to have to worry about the correlation between parameters when conducting statistical analysis.<br>I was not expecting such a high level of accuracy when I actually used AI, and the accuracy has been visibly improved by increasing the amount of training data. Kawajiri: What I have been feeling in recent years is that the data is very useful.
In recent years, I feel that although AI tools are very advanced, the know-how of the human side in using AI cannot be taught as a textbook.
Well, through various exchanges with Mr. Kawajiri, I have come to realize that there is a part of know-how that I was not expecting. I think it would be good if there is a set of tools, and if there is a way to learn how to create detailed data, how to handle calculations, etc., as well as how to use and learn such know-how.
What are your expectations for us and Multi-Sigma in the future?
I think Multi-Sigma is a very easy-to-use, no-code analysis tool, and I would like to continue to use it. We will continue to use Multi-Sigma as it is a no-code and easy-to-use analysis tool. When they hear the word “AI,” some people may think that it is difficult to use or difficult to use, so I think that if the tool can be used easily by any user, the number of users will increase.
Thank you very much. We would like to make the tool even easier to use and analyze, including the UI. Thank you very much for taking time out of your busy schedule to be interviewed.
