Solution
Material Science Solution
AI is considered a very powerful tool for the materials science field. This presentation will introduce the challenges in the industry and the benefits of applying Multi-Sigma™.
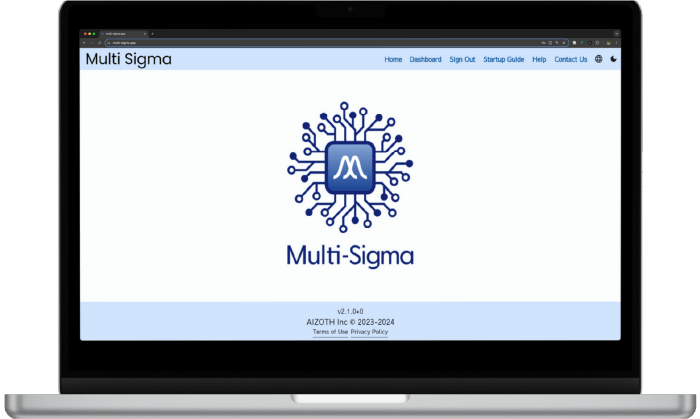
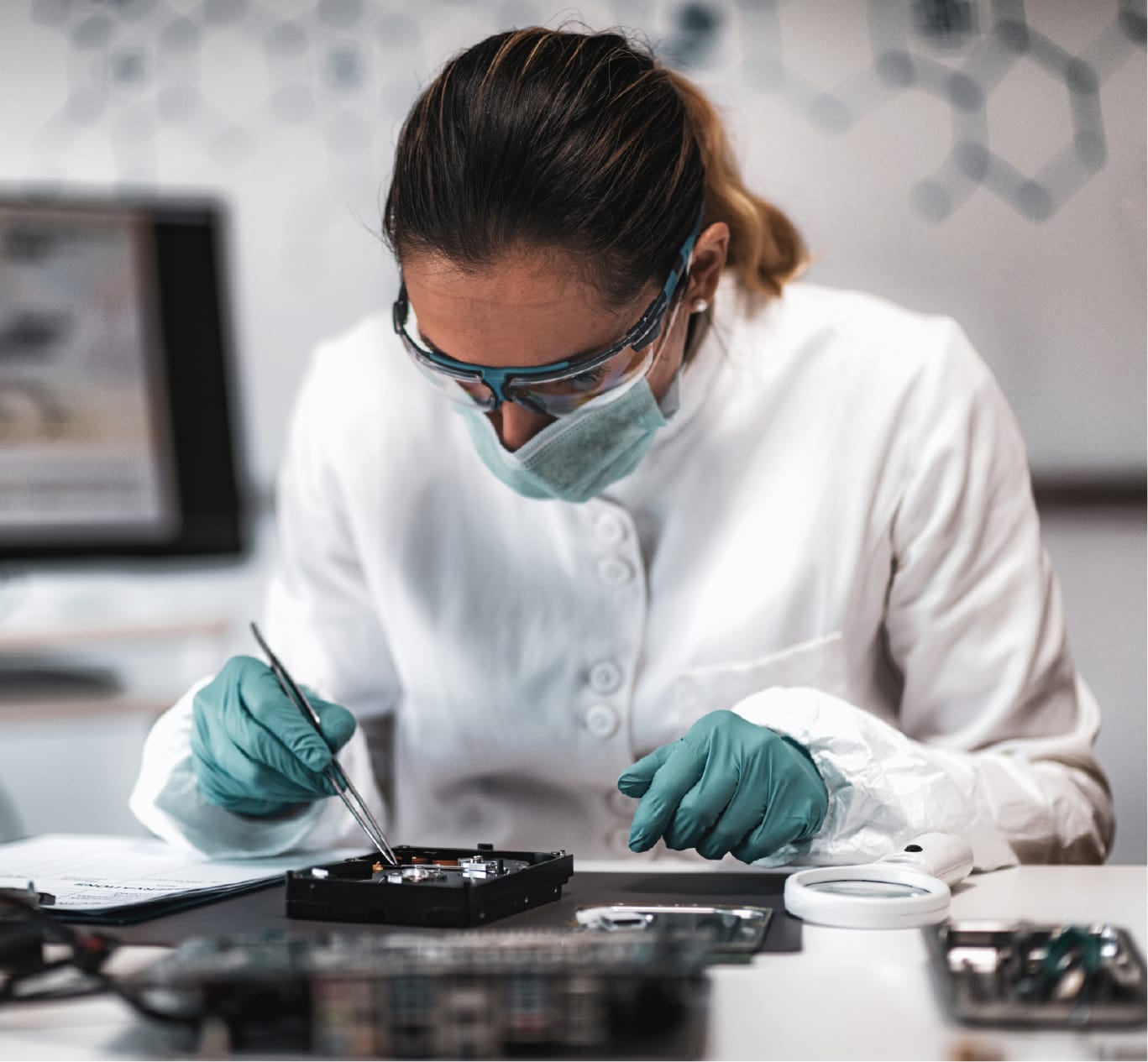
Challenges in the materials science industry
Lack of data and bias
Lack of high-quality experimental data and bias toward specific materials and conditions pose significant problem in the versatility and accuracy of trained AI model.
Treatment of diverse materials
Designing advanced materials involves complex, unique interactions between composition and synthesis conditions. Mapping these black-box interactions between control variables and material properties remains a significant challenge.
Limitations of Computational Resources
Materials simulations using molecular dynamics and quantum chemical calculations require substantial computational resources. While AI-based prediction and optimization can address some issues, building a generalizable model and tuning hyperparameters remain time-consuming tasks.
Interpretation of results
Interpreting AI model outputs is essential in materials science, as understanding why predicted results occur enhances their practical utility. Black-boxed results reduce usefulness, so developing methods for explaining AI outputs is crucial for real-world acceptance and application.
What Multi-Sigma™ can do for the materials science industry
Start with minimal data
Multi-Sigma™ can build AI models using small amounts of data. Even with biased datasets, it achieves high-accuracy predictions and optimization through its unbalanced data adjustment function.
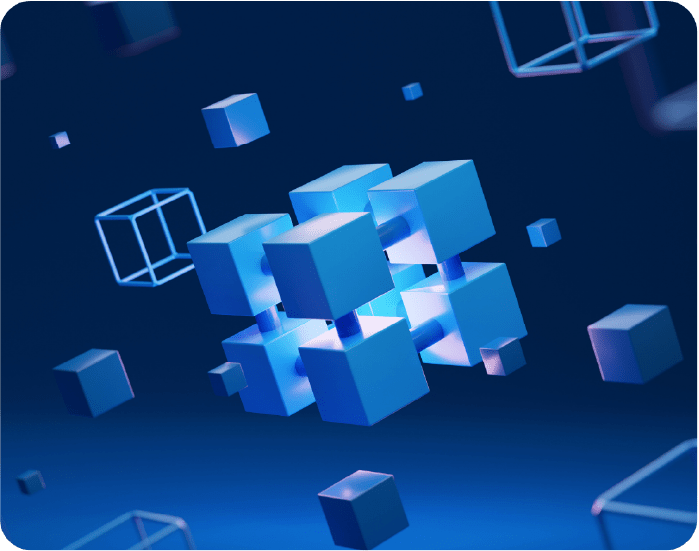
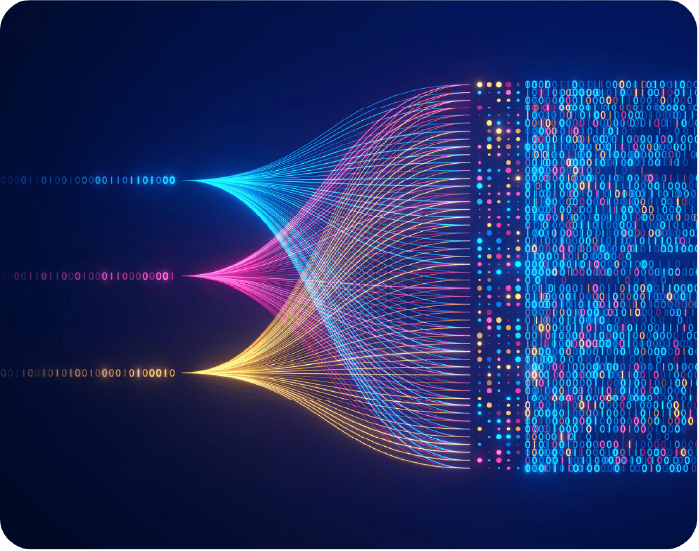
High versatility
Multi-Sigma™ is a powerful tool for numerical analysis across various material types. With numerical data on input and output parameters of materials design or synthesis, it can model black-box interactions to provide realistic solutions.
Simulation resources can also be used effectively
Multi-Sigma™’s AI model acts as a surrogate by creating simplified versions of complex simulations. These surrogate models enable rapid screening and optimization, maximizing the utility of available simulation resources.
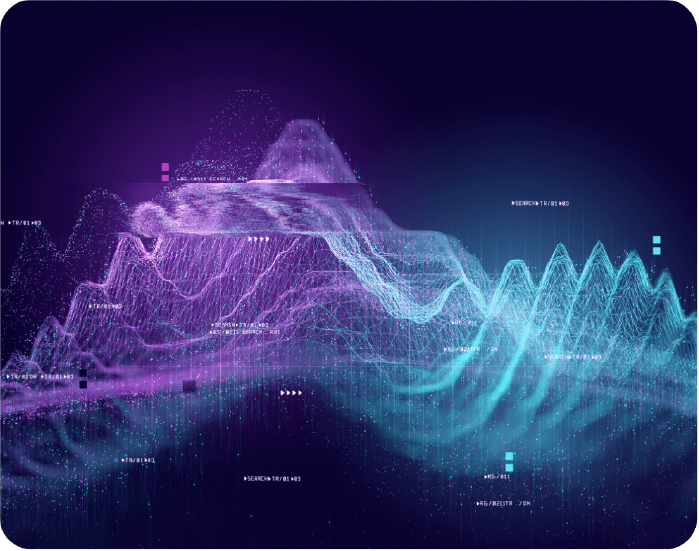
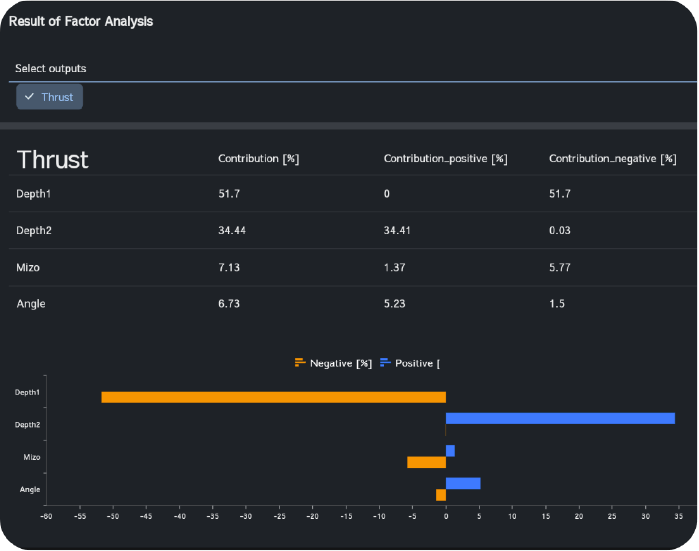
Factor analysis function enables white-boxing
Multi-Sigma™’s “factor analysis” function enables white-boxing by quantitatively describing how inputs affect outputs. This allows users to understand the rationale behind the outputs and interpret results effectively.
Advantages of Multi-Sigma™
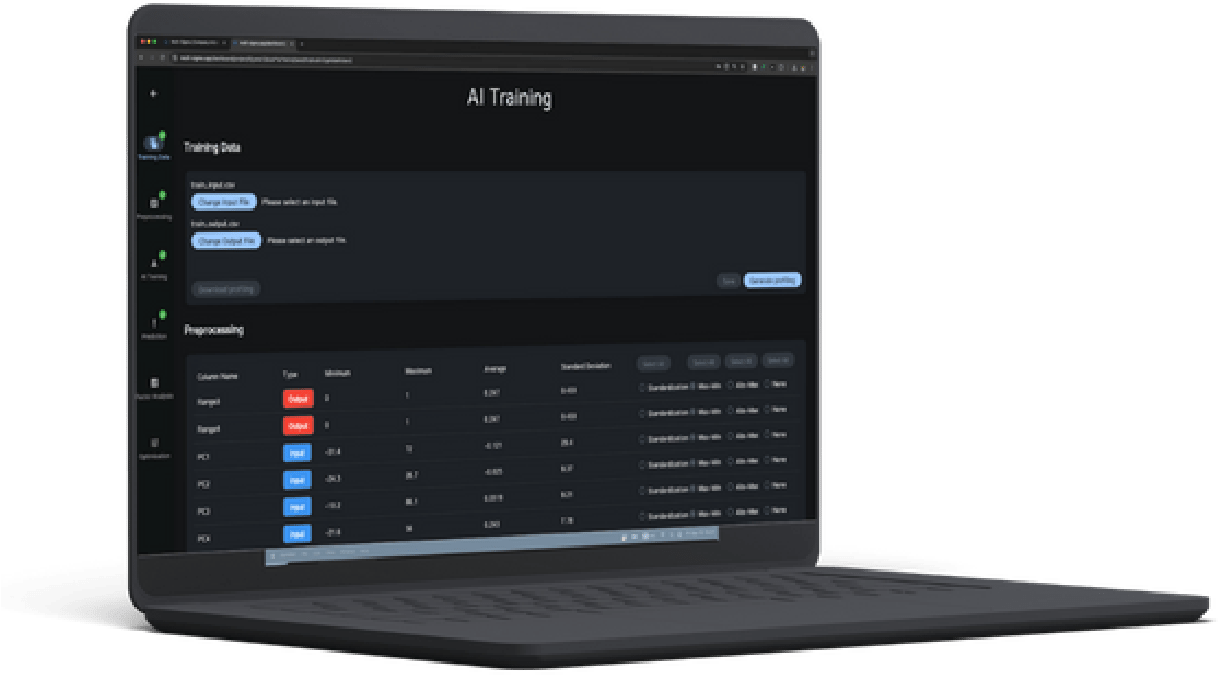
Deep learning with minimal data
Multi-Sigma™ enables modeling and analysis from small experimental datasets, reducing experimental effort and shortening R&D cycles across various fields.
High-precision prediction
Offering high-accuracy predictive, factorial, and optimization capabilities, Multi-Sigma™ allows for realistic and effective analysis based on experimental data.
Multi-objective optimization
Capable of exploring up to 200 inputs to satisfy 100 outputs, Multi-Sigma™ finds optimal solutions for multiple competing goals simultaneously, aiding in new material development and improving manufacturing efficiency.
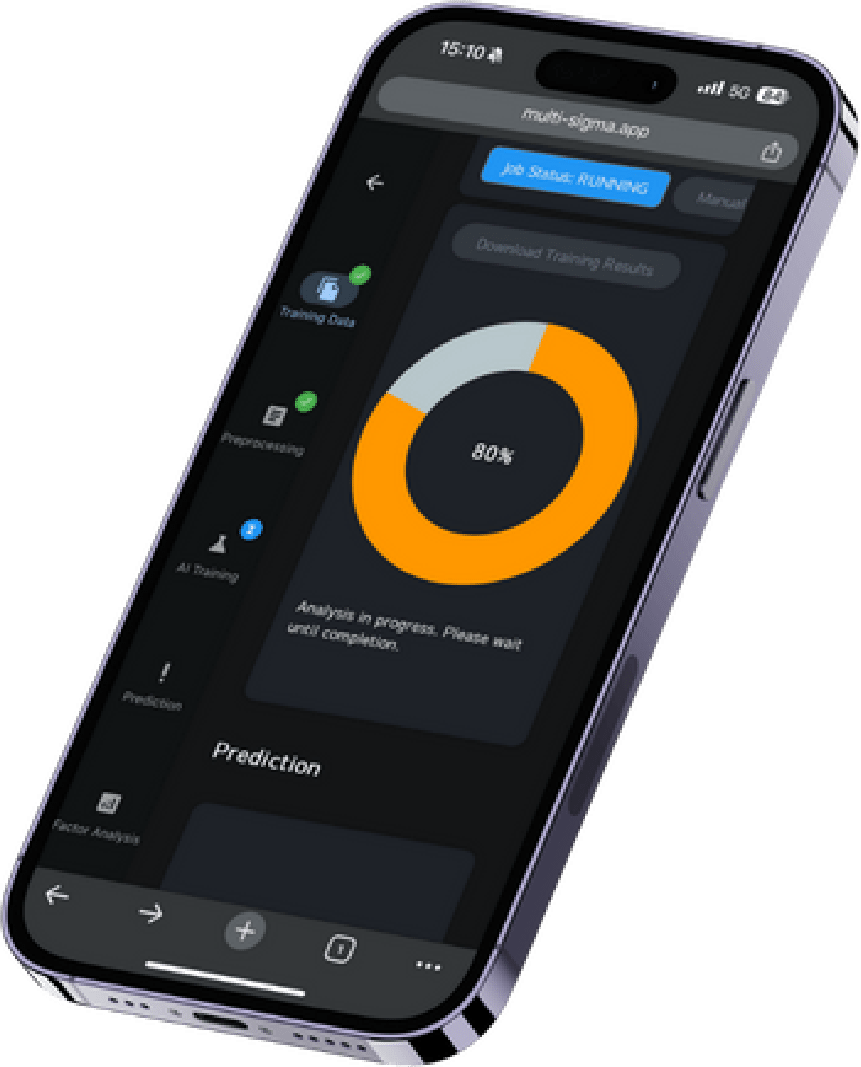
Explainable AI (xAI)
Multi-Sigma™ includes a factorial analysis function that reveals which inputs affect which outputs, their extent, and whether the impact is positive or negative, enabling users to interpret results and understand internal mechanisms.
No-code and cloud-based
Requiring no programming skills, Multi-Sigma™ provides an intuitive interface for data analysis with just a few clicks. As a cloud-based tool, it continuously updates with new features and bug fixes based on user feedback.
Chain Analysis
By linking inputs and outputs across multiple processes, Multi-Sigma™’s chain analysis function chains multiple AI models for comprehensive analysis, enhancing AI performance and revealing process mechanisms.