AIZOTH Inc. exhibited at Materials Research Society (MRS) Fall Meeting & Exhibit, held over three days from Tuesday, December 3, to Thursday, December 5, 2024, at the Hynes Convention Center and Sheraton Boston Hotel in Boston, USA.
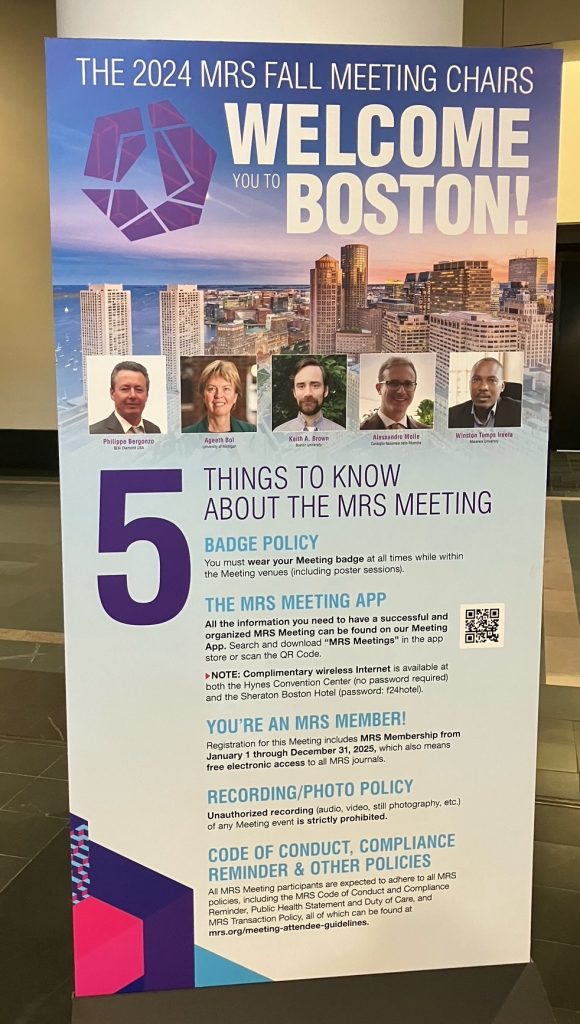
At the MRS Fall Meeting & Exhibit on December 4, we presented Multi-Sigma—An Easy-to-Use AI Analysis Platform for Prediction and Optimization and a workshop titled Multi-Sigma—An Integrated AI Platform for Simultaneous Prediction and Optimization of Multiple Target Objectives. Researchers and developers who expressed interest in our AI analysis platform, Multi-Sigma®, during these sessions later visited our exhibition booth as well. Additionally, over 130 attendees visited our booth between December 3 and 5, engaging in discussions on various topics, including demonstrations of Multi-Sigma’s features and use cases, as well as exchanging ideas on data analysis.
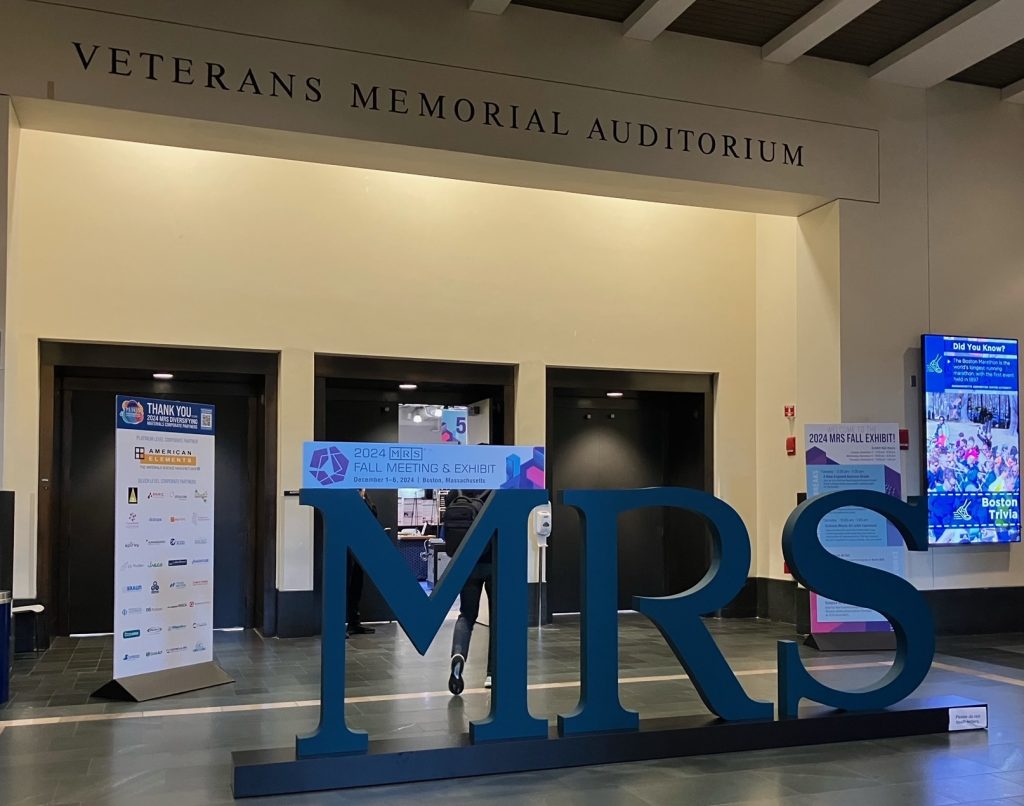
Researchers working on semiconductor experiments have expressed interest in leveraging AI models to analyze data collected from repeated experiments conducted under varying conditions to observe semiconductor characteristics. They also inquired about the number of experiments required to build such AI models effectively. In response, we introduced our platform, Multi-Sigma®, which enables the development of high-accuracy AI models based on neural networks using such experimental data. We further highlighted Multi-Sigma’s Auto-Tuning feature, which automatically tunes neural network hyperparameters. This innovative functionality significantly reduces the number of experiments needed to build an AI model, making it possible to achieve accurate results with as few as a few dozen experiments.
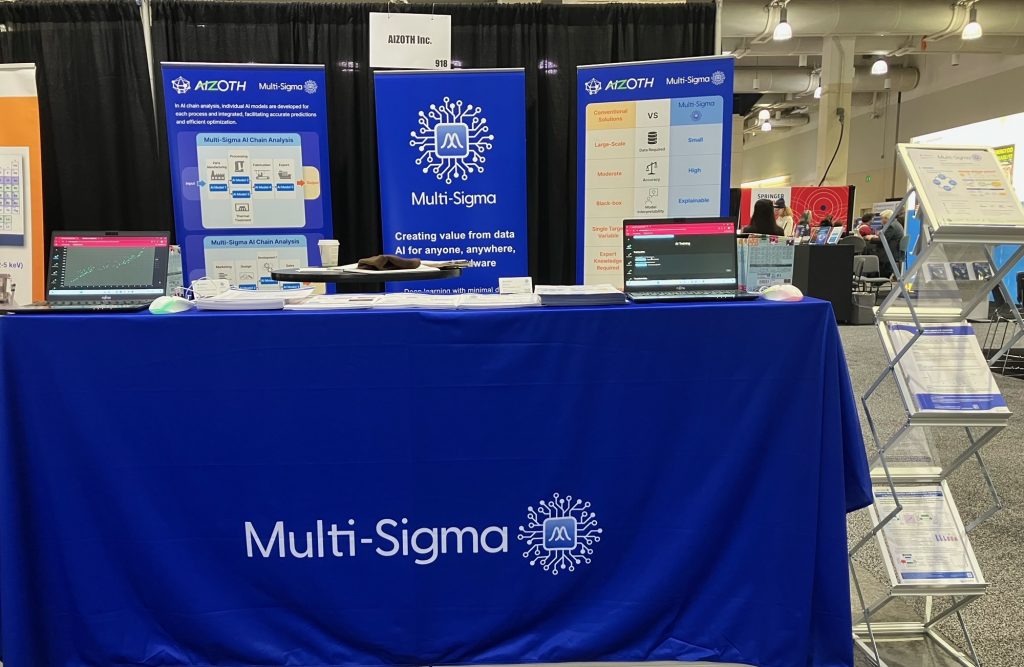
Researchers and developers utilizing molecular dynamics (MD) and density functional theory (DFT) simulations have approached us with concerns about the significant computational time required for these simulations. They inquired about the possibility of leveraging AI to enhance efficiency. In response, we introduced examples of surrogate models or emulators that combine simulations with machine learning. These surrogate models use AI to learn from the input and output data of previous simulations, enabling them to predict and substitute simulation results for unknown inputs. Additionally, we presented specific examples of surrogate models built using our Multi-Sigma® platform and discussed how these models could be applied to the research of those who visited our exhibition booth. Through these discussions, we explored potential applications of this approach to address their computational challenges.
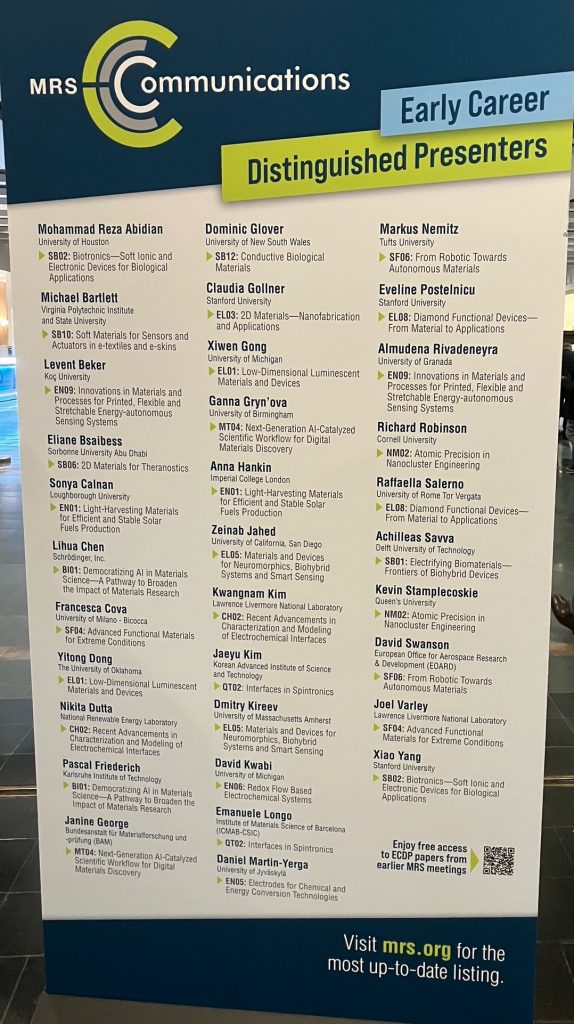
At the exhibition, we introduced case-studies utilizing Multi-Sigma®, including analyses of (1) Prediction Model Integration and Multi-Objective Optimization in Pharmaceutical Development, (2) Hydration Free Energy Prediction in Molecular Design, and (3) Balancing Target Density Control and Performance Maximization in MOF Development. These analyses employed Multi-Sigma’s AI Chain Analysis, factor analysis, and multi-objective optimization functions for data analysis. The case-study leaflets were well-received for providing clear and practical examples, helping visitors envision how Multi-Sigma could be applied to their research and development. In addition to the leaflets distributed at the exhibition, other materials are available for download from this page. If you have any questions about our products or consulting services, please feel free to contact us using the information below.